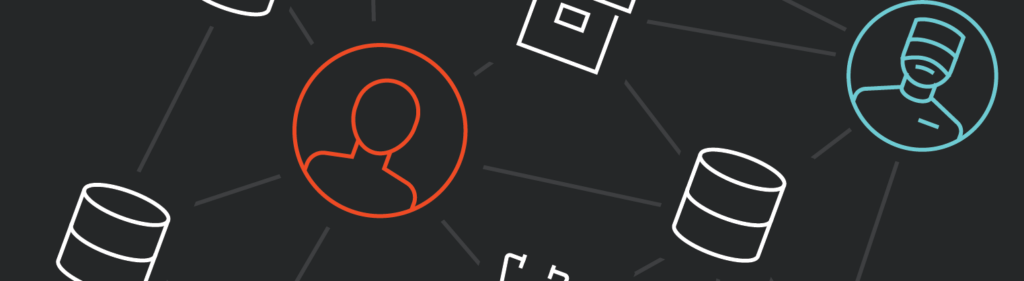
Healthcare is in a state of precarious flux. With COVID-19 forcing payors and providers to implement new tools in mere months — when it previously would have taken years — digital transformation has become a critical factor in adjusting to a new normal. Additionally, patient experience has become a top priority as patients, or consumers, demand seamless digital experiences.
As these tools range from telehealth to remote access of electronic medical records (EMR) data, the importance of interoperability between the clinic setting and digital interfaces has never been higher. As patients interact with digital products more than ever before, the healthcare industry has shifted the data to become patient-centric versus provider-centric. In addition, the sophistication that patients demand from healthcare tools is a call to arms to improve the patient experience using a well-implemented data interoperability strategy to empower this digital transformation.
Data silos more prevalent than ever
Historically, providers have primarily held all patient data through an electronic medical records (EMR) system. As the needs for data interoperability have evolved, the data has shifted from EMR-centric to the demand for availability within more applications and digital products. With more fragmented data than ever before, it has put a burden on the providers who are responsible for reconciling the differences and attempting to create a cohesive interaction point for patients. Frameworks like Health Level Seven (HL7) and Fast Healthcare Interoperability Resources (FHIR) have standardized the data pipelines to help providers interact with external parties.
The chart below shows how the shift in data access has evolved:
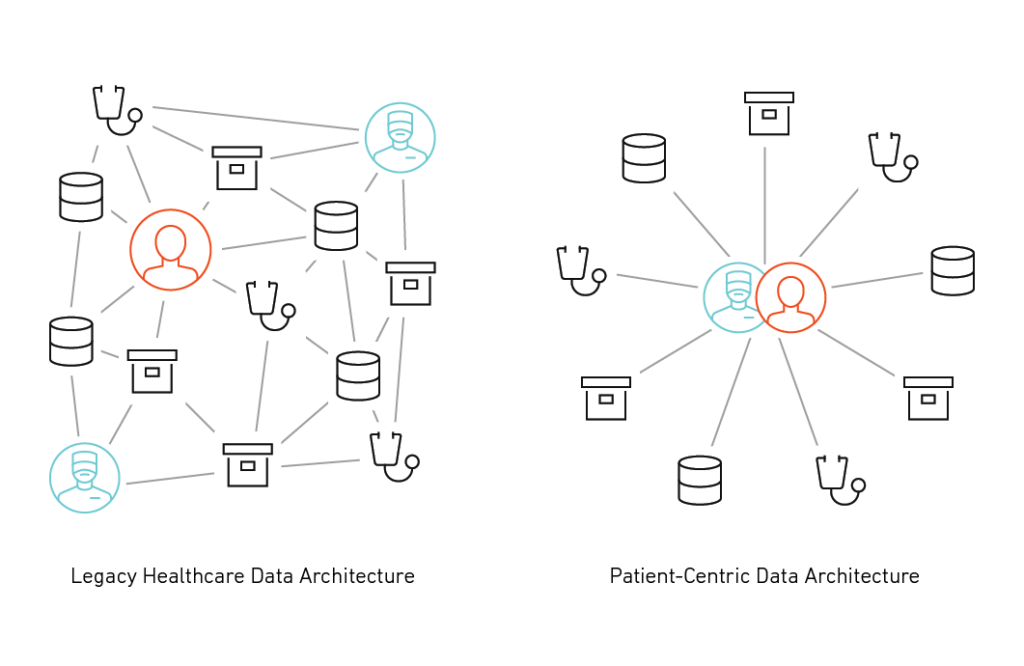
Emerging digital tools
Digital products from telehealth to clinical chart access have given unprecedented touchpoints to a patient’s previous in-clinic visits. As the digital footprint increases, the data available from these applications also increases. Organizations can now gain a view into how engaged a patient is in their care, and this data is incredibly valuable as providers create more patient-level care pathways versus an aggregate population view. Providers can maintain a more intimate relationship with patients while understanding how they respond to more personalized care recommendations.
While intimate relationships are vital to a well-orchestrated patient experience, there needs to be protection for patients to ensure data is not shared or used without explicit permission. Two landmark initiatives — the Cures Act and the MyHealthEData Initiative2 — are starting to help ensure patients have more control over their data.
Interoperability gives to agnostic solutions
Part of the slowness in leveraging the rich data these digital tools are producing is caused by providers often not having a centralized data repository. Historically, the EMR was the centralization for patient data, but it’s no longer a solution for housing the many types of new patient data. Cloud providers like Amazon Web Services (AWS), Google Cloud Platform (GCP), and Microsoft Azure have risen to the challenge by offering robust solutions that offer not only storage, but also the ability to analyze and integrate back into nearly any environment. Nearly one-third of hospitals and health systems reported low interoperability success, even within internal applications, according to a survey from the Center for Connected Medicine (CCM).1
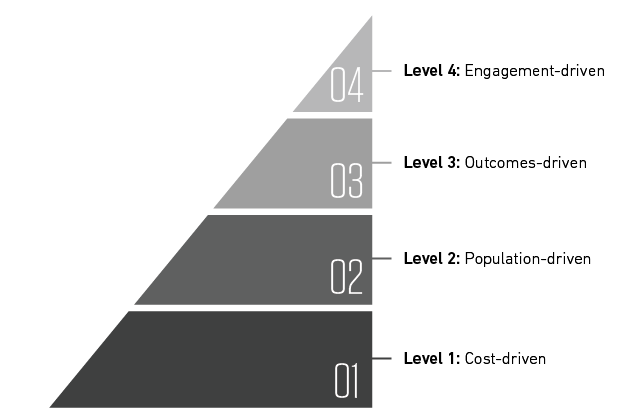
The four levels of data maturity to enrich the patient experience
As healthcare organizations evolve, their maturity in patient experience must also evolve:
Level 1: Cost-driven
Often, revenue and cost metrics drive the need for a sound data interoperability strategy. Whether it’s a claims clearinghouse or pharmacy integration, this is the first level in interoperability. Healthcare costs are very confusing for patients due to insurance responsibility, copay, or the plan’s deductible. One successful example of this is the Medicare Blue Button 2.0, which attempted to centralize data sharing for a patient’s Medicare Part A, Part B, and Part D claims and encounter data.
Level 2: Population-driven
Population health management has been a vital component in providers helping their patients more effectively through aggregations of patient data. An example might be providers looking at the entire diabetes group of patients and finding trends to help them care for this population more effectively. With the rise of Accountable Care Organizations (ACOs), providers can look at the population of patients and help identify trends to be able to better treat the condition — and therefore the patient as a whole. Of course, there are many behavioral differences in patients, but ACOs can identify which patients are non-compliant or are at-risk for progressing their existing condition or developing a new chronic condition.
Level 3: Outcomes-driven
Outcomes help the organization provide context if a treatment or therapy is positive or negative for a patient. The glaring issue is that there are care pathways for treatment, but there is seldom agreement on what qualifies as a “positive” outcome. Doctors who specialize in a specific treatment, disease or condition lead the charge to establish the benchmark for their respective research fields. Bundled payments have been a transition step to value-based-care methods.
Level 4: Engagement-driven
The final stage of data maturity is when applications, both internal and third-party, are not only working harmoniously, but can leverage each application to improve the other. For example, a scheduling application can give insight to recommend a time window for the patient while in the clinic. This example would be pulling in a scheduling app and identifying when the previous patient’s appointments were scheduled. This data can sync into the EMR and identify if the findings were severe, or if the next appointment is a routine appointment. Next, leveraging machine learning, the clinic would optimize when most no-shows occur and find times that appointments are held. Recommendation can be clinic-specific to optimize how many patients can be seen and also, when the patients personalized recommended windows occur. Finally, after appointment booking takes place, the appointment is entered automatically into a patient communication mechanism to give timely reminders and pertinent updates to help alert the patient. If the patient does not view or confirm the appointment, an algorithm can send a text asking if the patient would like to confirm or reschedule at their earliest convenience. This loop creates a small touchpoint saying it’s okay to move the appointment, as the provider is shifting the importance away from the appointment and towards the patient’s busy lifestyle.
So, where do organizations start on this journey of patient-centric analytics to power the healthcare organization? Before investment in new tools occurs, it’s vital to document existing data sets and their dependencies. Time after time, organizations implement new tools, only for them to not be fully adopted and, as a result, lowering ROI. The stakes couldn’t be higher; an independent study estimates savings of $77.8 billion per year of protection to health systems3.
Data is not just another component to implement for health systems and infrastructure. If done correctly, it will act as the interstitial tissue to empower all systems to work more efficiently and ultimately improve patient outcomes.